Quantitative Estimation of Surface Soil Moisture in Agricultural Landscapes using Spaceborne Synthetic Aperture Radar Imaging at Different Frequencies and Polarizations
Volltext
Abbildung
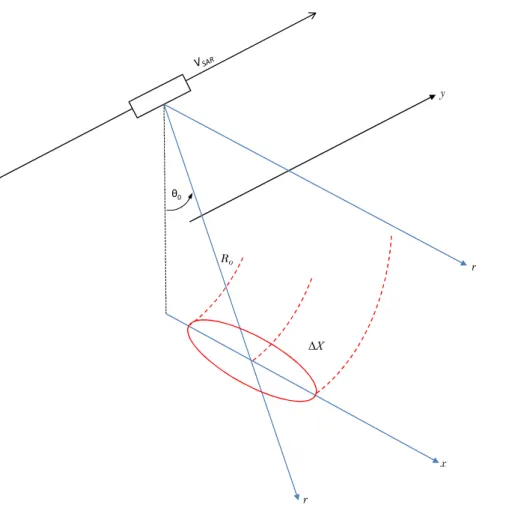


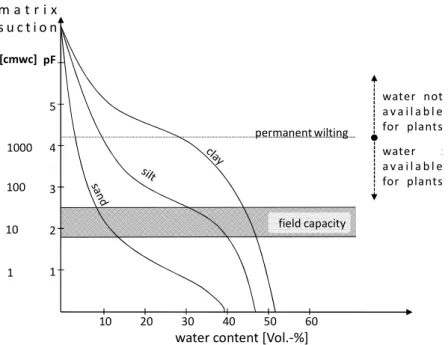
ÄHNLICHE DOKUMENTE
TA B L E 3 Effects of species traits on species geographic range size, climatic niche size, mean cover and skewness of cover values.. In addition, the axes captured some
Along with soil CO 2 efflux the parameters temperature and soil moisture were measured weekly and a soil survey analysis was conducted in 2009, including soil bulk density, root
We explore the issue as to whether soil moisture initialization is relevant for decadal predictions with focus on extreme periods which has not been attempted in this time scale up
ATI model does not meet the requirements of precise soil moisture retrieval. Real
While masking based on the SSM sensitivity was always possible, the masking based on fraction of open water bodies was subject to spatial cover- age of the Permafrost
ASCAT SSM product is the result of an im- proved SSM retrieval algorithm developed at the Institute for Photo- grammetry and Remote Sensing (IPF) of the Vienna University of
Supplementary Figure S2: Soil moisture at 10 cm below surface [ % (percentage of volumetric water content)] and soil temperature at 10 cm below surface (°C) over the Experiment
The objectives of this paper are: (i) to study the impact of soil moisture and temperature on the net ecosystem carbon balance and its components in two consecutive