Electrophysiological signature of conscientiousness during task performance
Volltext
Abbildung
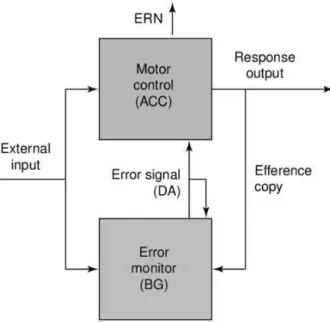
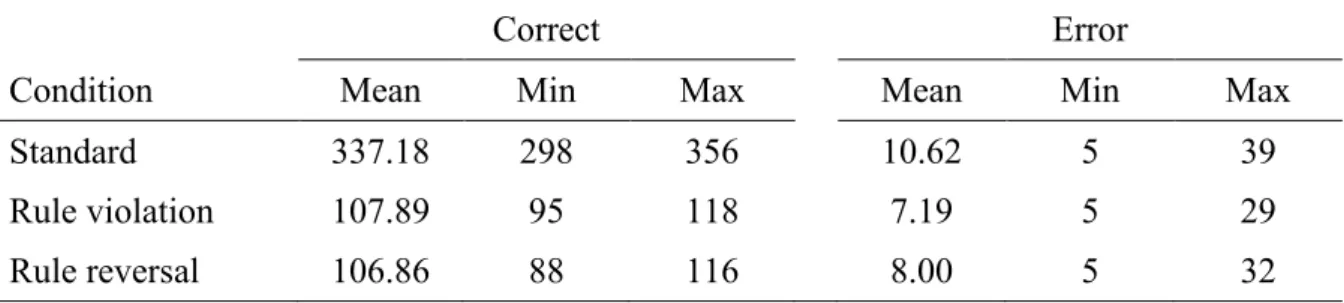

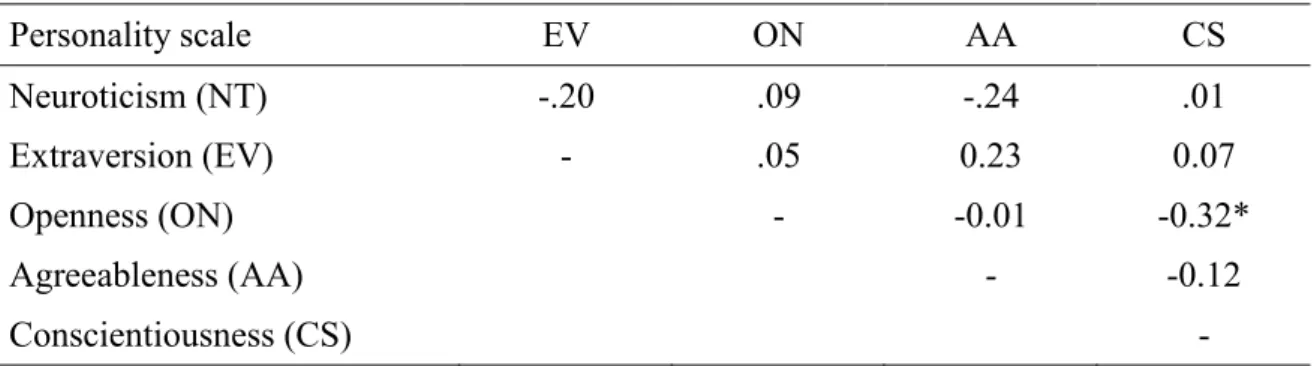
ÄHNLICHE DOKUMENTE
Because no temperature data when samples were taken from bottles are recorded, it was assumed that the density of samples is 1022.156 kg/m3 (which assume the temperature for 28
This paper reports preliminary results from measurements of frozen ice samples, the achievable signal intensities, standard deviations and calibration graphs as well as the
Both trait impulsivity scales, functional and dysfunctional, have significant negative correlations with high school student grade point averages, except for Sciences GPA
[r]
Guangdong Meteorological Observation Data Center, Guangzhou, China.. Funding information was missing in the
With this and the fact that users only exchange ratings made by themselves, we conclude that if u a exchanges ratings while consuming items, the local set of rating tuples
Besides this effect of task type on P300 amplitude, both Novelty P3 and P300 amplitudes were sensitive to matrix size such that larger matrices (and hence
Eldar Khan, the usurper Shamkhal, the Usmi and Sorkhey of the Qazi-Qomuq gathered in Qazanesh with the intention to attack Khass Bulat Khan, the Shamkhal, 106 but they were