A Study of Biomedical Time Series Using Empirical Mode Decomposition
Volltext
Abbildung
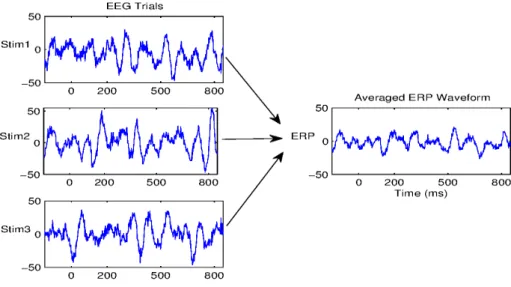
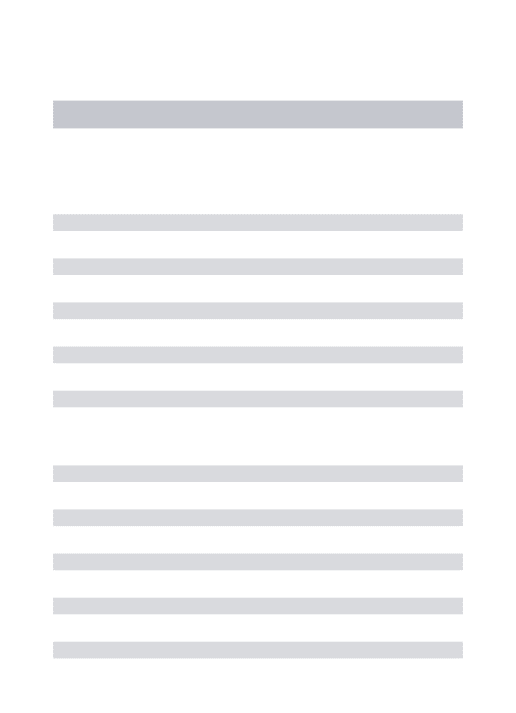
![Figure 4.1 Examples of Gestalt rules for perceptual grouping (adapted from [166]).](https://thumb-eu.123doks.com/thumbv2/1library_info/3945228.1534145/75.892.196.725.153.676/figure-examples-gestalt-rules-perceptual-grouping-adapted.webp)

ÄHNLICHE DOKUMENTE
As we can see in Figure 4, the results showed the feasibility of the proposed method as a temporal segment selection strategy feature to increase multi-class classification
We instructed subjects to execute hand open/close, supination/pronation, and elbow extension/flexion movements according to cues presented on a computer screen, i.e., 6
Considering different DL architectures, we investigated deep neural network (DNN) modules, which are consists of several fully-connected layers; a proposed hierarchical DNN
Two conditions are simulated in this way while the amount of vertical dis- parity in one condition (3D-2) is 40 % less than the other condition (3D-3). The cube is presented to
The results confirm that our method is able to significantly reduce different types of artifacts like, e.g., high frequency muscle artifacts or low frequency artifacts caused by
The results confirm that our method is able to significantly reduce different types of artifacts like, e.g., high frequency muscle artifacts or low frequency artifacts caused by
Department of Mathematics, Imam Khomeini International University, Ghazvin, 34149-16818, Iran Reprint requests to S.. Some numerical results are given to demon- strate the validity
In this paper the hamotopy analysis method (HAM) is used for solving ordinary differential equations which arise from problems of the calculus of varia- tions.. This approach