Advancing Functional Understanding of Primary Production in Drylands: Insights from a Data-Integration Approach
Volltext
Abbildung


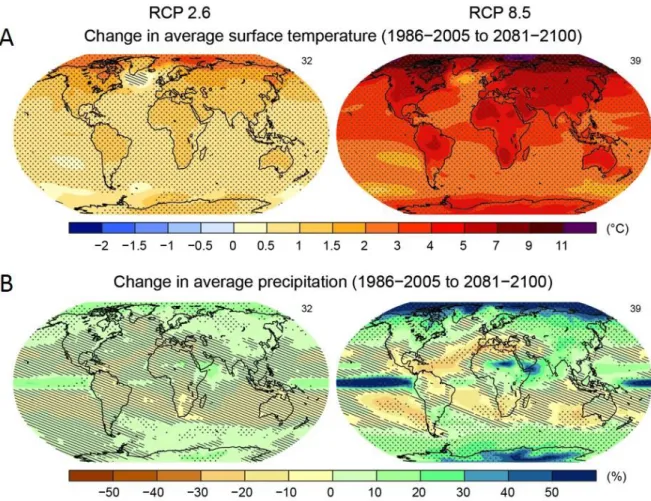
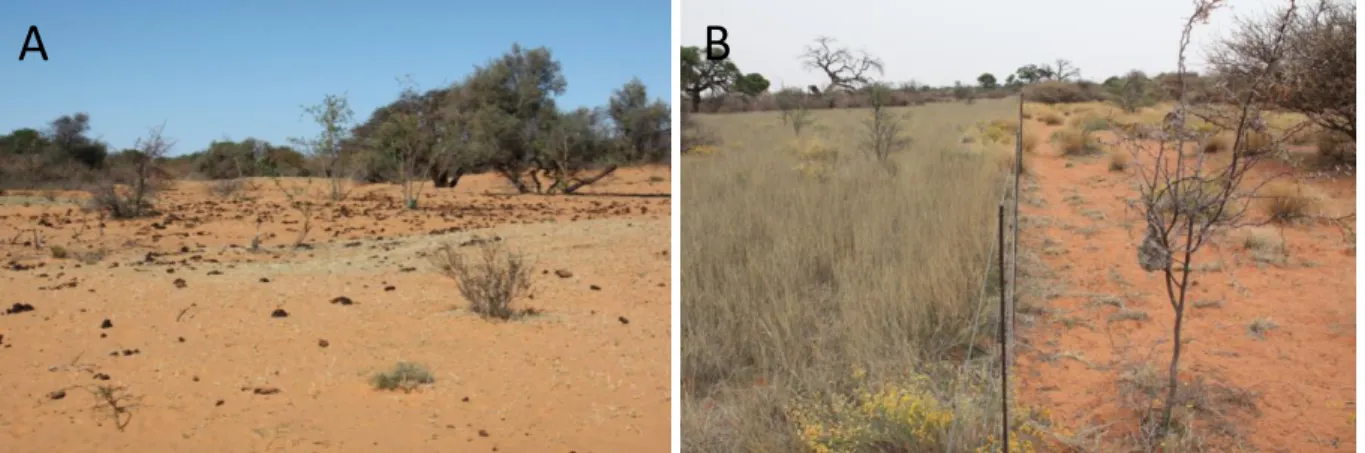
ÄHNLICHE DOKUMENTE
The goal of this study is to quantitatively estimate the seasonal course of daily gross primary production (GPP) of crops with the model PIXGRO, achieving critical parameterization
It’s easy to understand New Delhi’s reluctance to support security‑focused institution building in the IOR that it can’t control. The strategic architecture in the IOR is very
For catch-bonds, bond lifetimes are initially increased to a certain threshold (orange). Catch-bonds are accompanied by a change in binding site conformations. Two
At the same time, as mobile internet becomes available and affordable, the mobile device can play as personal access point to private and shared data, as well as applications, which
A production method, that ensures good pollen quality is described, as well as the main quality criteria, that can be included in a future standard.. There are
Luminance uniformity, uniformity of reflectance, uniformity of luminous flux, contrast uniformity or colour uniformity depends on the technology (use of the equipment) as well as
We outline a methodology of a full and verified carbon account of terrestrial ecosystems (FCA) that supposes unbiased assessment of relevant proxy values (here: Net Ecosystem
For each species the following informations are provided in German and English: taxonomy (species name, author, quotation of the original description, in some cases