Theory and Applications
Volltext
Abbildung
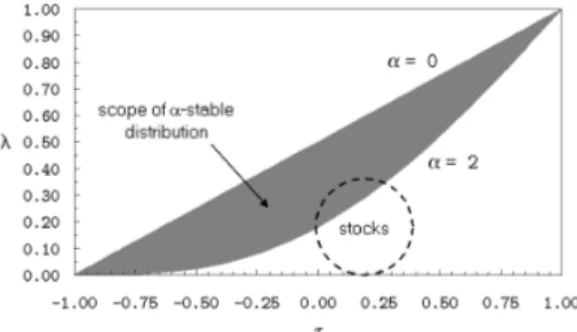

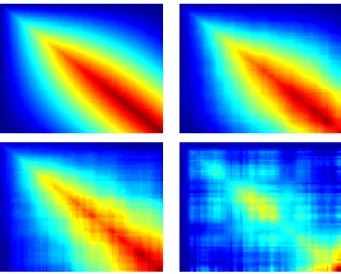

ÄHNLICHE DOKUMENTE
The reduction in the variance due to orthonormal variates is appreci- able, but even t h e crude estimator gives acceptable results.. After some reflection i t
Besides the pure technical features, the usability of a PKI- enabled application plays a crucial role since the best security application will fail in practice if its usability
We take the theorem of Lindner and Seidel, that relates the P -essential spectrum of a band-dominated operator with the spectra of its limit operators and was mentioned in
Key words: Copper Ion Complexes, Potential Energy Surface, Gas Separation, Binding Energies, NBO Analysis,
Since the elements of the Chirgwin-Coulson bond order matrix are introduced directly as variational parameters for the electron density instead of just being used for the
A main motivation for the use of mixed autoregressive moving average models is to satisfy the principle of parsimony. Since stochastic models contain parameters whose values must
Many properties and concepts like, e.g., Dirichlet problem, meanvalue property, maximum principle, fundamen- tal solutions, Perron’s method, Dirichlet’s principle, spectra, etc.,
In Section 4 we investigate the asymptotic behaviour of the diameter of the set of points that form the Poisson point process with intensity n κ , where κ is a spherically